AI tool detects Parkinson's up to 15 years early with 96% accuracy
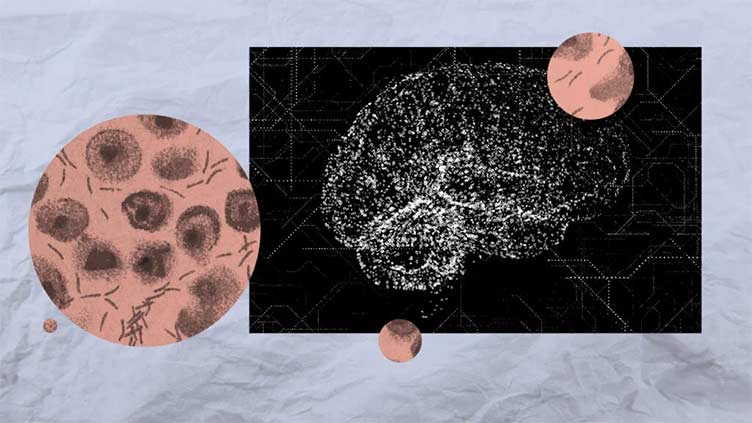
Around 90,000 people in the United States are diagnosed with Parkinson's
ISLAMABAD, (Online) - Parkinson’s disease is a neurodegenerative condition characterized by unintended movements such as shaking, stiffness, and difficulty balancing. It is caused by the loss of nerve cells in the brain, leading to reduced levels of dopamine, which plays a key role in movement.
Around 90,000 people in the United States are diagnosed with Parkinson’s, and over 10 million people live with the condition worldwide.
Parkinson’s is the second most common neurodegenerative condition after Alzheimer’s disease, and cases are growing more rapidly than other neurological conditions.
Currently, Parkinson’s is diagnosed based on symptoms, medical history, and a physical examination. There are no tests for Parkinson’s.
Tests that can detect Parkinson’s could improve care and management strategies for the condition.
Recently, researchers developed an AI tool that can predict signs of Parkinson’s from patients’ blood samples up to 15 years before symptom onset.
“This tool will be a game changer for Parkinson’s diagnosis by providing an objective, reliable, and highly accurate way to predict conversion in patients at risk of developing the disorder,” Dr. Mya Schiess, neurology professor and director of the Movement Disorders & Neurodegenerative Diseases Program at UTHealth Houston, who was not involved in the study, told Medical News Today.
The study was published by the American Chemical Society.
More accuracy in diagnosing Parkinson’s
For the study, the researchers gathered healthcare data from 78 individuals from Spain. Each participant provided a blood sample between 1993 and 1996 and was followed for 15 years. Among the participants, 39 eventually received a diagnosis of Parkinson’s, whereas 39 did not.
The researchers next utilized an AI tool called CRANK-MS to analyze the data. In particular, they compared different combinations of metabolites—chemicals the body creates when breaking down food, drugs, chemicals, and its own tissue—among those who developed Parkinson’s and those who didn’t.
“Typically, researchers using machine learning to examine correlations between metabolites and disease reduce the number of chemical features first, before they feed it into the algorithm,” W. Alexander Donald, associate professor in the School of Chemistry at the University of New South Wales Sydney, Australia, one of the study’s authors, said in a press release.
“But here, we feed all the information into CRANK-MS without any data reduction right at the start. And from that, we can get the model prediction and identify which metabolites are driving the prediction the most, all in one step. It means that if there are metabolites which may potentially have been missed using conventional approaches, we can now pick those up,” he added.
Using CRANK-MS, the researchers identified metabolite combinations unique to participants who developed Parkinson’s. They identified 96% of people who developed Parkinson’s cases within 15 years from these combinations alone.
The researchers noted that their new tool was more accurate in diagnosing Parkinson’s than existing clinical assessments by movement disorder specialists, which have an accuracy of 80%.
The researchers also reported that CRANK-MS could also diagnose Parkinson’s 84.3% of the time from skin sebum samples when they tested the algorithm with a second cohort of 274 NHS patients.
AI tool may improve care for Parkinson’s
MNT spoke with Daniel Truong, neurologist and medical director of The Parkinson’s and Movement Disorder Institute at MemorialCare Orange Coast Medical Center, who was not involved in the study, about how CRANK-MS could improve Parkinson’s care.
“The AI tool demonstrated the ability to detect signs of Parkinson’s disease up to 15 years before the onset of symptoms, providing the opportunity for intervention and treatment at an earlier stage,” he noted.
He continued that the tool’s high level of accuracy could also allow potentially reliable diagnostic tools for early identification of risk factors and that the tool may have the potential for diagnosing other conditions too.